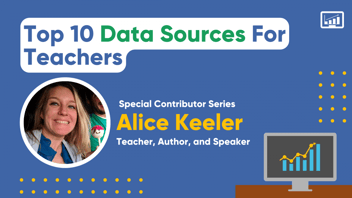
Published: July 12, 2022
Data has always been an essential part of classroom instruction, enabling teachers to measure student progress throughout the year. From test scores to attendance records, measuring student performance is nothing new. However, modern education technology is advancing data-driven instruction methods in innovative ways, utilizing data analysis to track the subtleties of student performance and refine classroom instruction.
Today's student data platforms empower teachers to provide data-driven instruction that maximizes student outcomes. With data-driven instruction, teachers gain a better understanding of learner progress and potential intervention methods. This allows them to optimize their instructional practice to improve individual student learning outcomes.
Whether you're new to data-driven instruction or you just need a refresh, this guide will help you understand the basics, from how it works to best practices.
Put simply, data-driven instruction is when educators collect and analyze big data on student learning and behavior to drive classroom instruction. While the phrase "big data" might sound scary, all it really alludes to is the scale and scope of the data you collect. Whereas traditional student data tracked macro-scale progress through summative assessments and standardized testing, big data incorporates daily classroom and assessment information for a detailed view of student performance.
Data-driven instruction is a continuous process in which educators gather student information, perform data analysis to identify weak spots, and make the necessary adjustments to their next lesson plan. While there are only three "steps" in this type of instruction, it's important to note that the process is continually repeated, creating a cycle of improved learning that constantly builds upon itself.
Data-driven instruction can be simplified into a three-step cycle of Acquisition, Analysis, and Action. Let's take a closer look at each of these individually to gain a better understanding of how they work together.
In the acquisition stage of data-driven instruction, educators gather information from formative assessments (e.g., small assignments like homework and quizzes) and summative assessments (e.g., standardized and cumulative test scores). These data sets might also include things like classroom teacher observations, attendance, student engagement, behavior, and home-life records. By collecting data from all of these different sources, educators and school leaders alike can gain valuable insights from a holistic view of student performance.
During data analysis, the teacher uses a student data platform to evaluate learning progress, looking for patterns at both the student and classroom levels. However, even with an intelligent education platform, knowing where to begin with big data can be overwhelming.
It helps to start with a single subject, focusing on the essential skills and key concepts that students need. From there, teachers can track individual student progress and aggregate classroom performance to help inform any future instructional decisions.
Finally, the action stage is when educators use the insights they gained to adjust and improve their next lesson plan to better accommodate student needs. By identifying specific learners that need more attention and uncovering areas of weakness, teachers can formulate differentiated instruction plans that promote mastery of any given subject. However, the journey isn't over yet. Teachers must also measure the efficacy of any instructional decision they make in the next round of assessments, leading right back to the first step of acquisition.
In some teacher circles, data has a history of being a dirty word, especially when emphasis is solely on test scores and scrutinizing teacher performance. However, data-driven instruction is quickly replacing that data uncertainty with data confidence as it streamlines the intuitive process teachers already go through to track and measure student progress.
By creating and distributing frequent and consistent assessments, teachers are able to continuously collect data on student performance. Insights drawn from data analysis then inform instructional decision-making, enabling educators to make continual improvements to their classroom activities, lesson plans, and student interactions. This process removes the element of blame from both teachers and students, producing a positive and supportive learning environment to maximize educational outcomes.
In addition to the obvious benefits for students and teachers, a culture of data-driven instruction can also be a huge advantage to school leadership and the district at large. By putting educators in charge of their own professional development process, the teacher and the student can learn from and adapt to each other, improving the efficacy of each lesson plan. This, in turn, improves overall student performance, demonstrating academic growth for the school or district beyond what standardized testing can show.
Lesson planning is an essential part of data-driven instruction, though the process may be vague or poorly outlined, especially for early-career teachers. Though there are unique student needs at each grade level, teachers can deploy a few universal strategies that use data to optimize lesson plans.
Formative assessment: Formative assessment is a continuous process in which educators monitor student performance to gauge understanding and improve instruction. For example, a high school teacher might have a daily quiz covering key concepts from the previous lesson plan. These assessments help teachers identify strengths and weaknesses in student comprehension to improve their lesson plans.
Summative assessment: A summative assessment evaluates student learning at the end of an educational unit. This can be a standardized test from the school district, a midterm exam or a final project. While summative assessments lack granular detail into student progress, they can provide context to overall academic growth.
Differentiated instruction: Using student performance data, teachers differentiate instruction to continuously match each student’s needs. Teachers can even group students by similar ability levels, academic interests, or learning preferences. That way, they can create targeted lessons, assignments, and unique learning avenues to match the strengths and struggles of each group in order to accommodate student needs.
Instructional scaffolding: This teaching method focuses on providing tailored support to specific students. Teachers can use student learning data obtained from regular and frequent formative assessments to identify where comprehension is falling behind. With this information, they can create a targeted approach for each individual student throughout the school year.
While these strategies can help outline general uses for data in the classroom, applying them can become confusing, especially for those who are new to data-driven instruction. To help you understand how teachers can use data to drive instruction, here are a few examples:
High school reading comprehension: Let's say you have a high school student who's struggling to understand the character journey in your current class novel. Using data from formative assessments like reflections and chapter quizzes, you can look back to see where comprehension started to fall off. You can then backtrack with the student to address what went wrong and get them up to speed quickly.
Middle school essay writing: If some in your middle school ELA class are still having trouble with usage of commas, colons, and semicolons, your previous writing assignments and rubrics may show a pattern of misunderstanding punctuation. With this information, you can plan to efficiently reteach punctuation, incorporating adjustments based on the confusion identified during data analysis. This whole-class adjustment will ensure a stronger grasp of grammar, empowering students to succeed throughout their educational journey.
Elementary school math skills: For upper elementary school teachers, you may have a group of students who do well in math but seem to struggle with long division. In this instance, you'll want to look at the data to see where there was a plateau in learning progress. These students perhaps do well with simple multiplication and division facts but have difficulty with multi-step arithmetic processes. With these insights, you can help transition this group into long division by introducing smaller numbers or multiples of two.
Additional at-home reinforcement: You can also use a student data platform to visualize and communicate performance information with parents. By showing parents precisely where their students are struggling, you can make them more aware of progress goals and learning objectives. Parents can then ensure students are working on take-home assignments for additional reinforcement.
Data can be intimidating—but with practice, data-driven instruction will become second nature. With data-driven instruction, teachers can leverage the power of big data for big results, optimizing lesson plans and instruction with student performance data to achieve accelerated learning outcomes. However, successful data-driven instruction requires an effective solution to help teachers acquire, analyze, and take action on student learning data.
Schoolytics is a powerful student data platform designed with administrators, parents, and, most importantly, teachers in mind. Our solution centralizes all of your class data into one location for easy, secure access. With real-time dashboards and efficient time-saving tools, you can maximize student learning outcomes without sacrificing productivity.
As a teacher, your number one job is to provide your students with the knowledge and instruction...